

Jacobian of Scalar Function The Jacobian of a scalar function is the transpose of its gradient. Of course, we usually integrate from $-2$ to $0$, not from $0$ to $-2$. The Jacobian matrix is invariant to the orientation of the vector in the second input position. What the Jacobian is, how it accounts for distortion, and how itĪppears in the change-of-variable formula.ĭefinition: The Jacobian of the transformation The distortion factor between size in $uv$-space and size in $xy$ This means that the Jacobian matrix contains vectors that help describe how a change in any of the input elements affects the output elements. Review: Change of variables in 1 dimensionīonus: Cylindrical and spherical coordinates
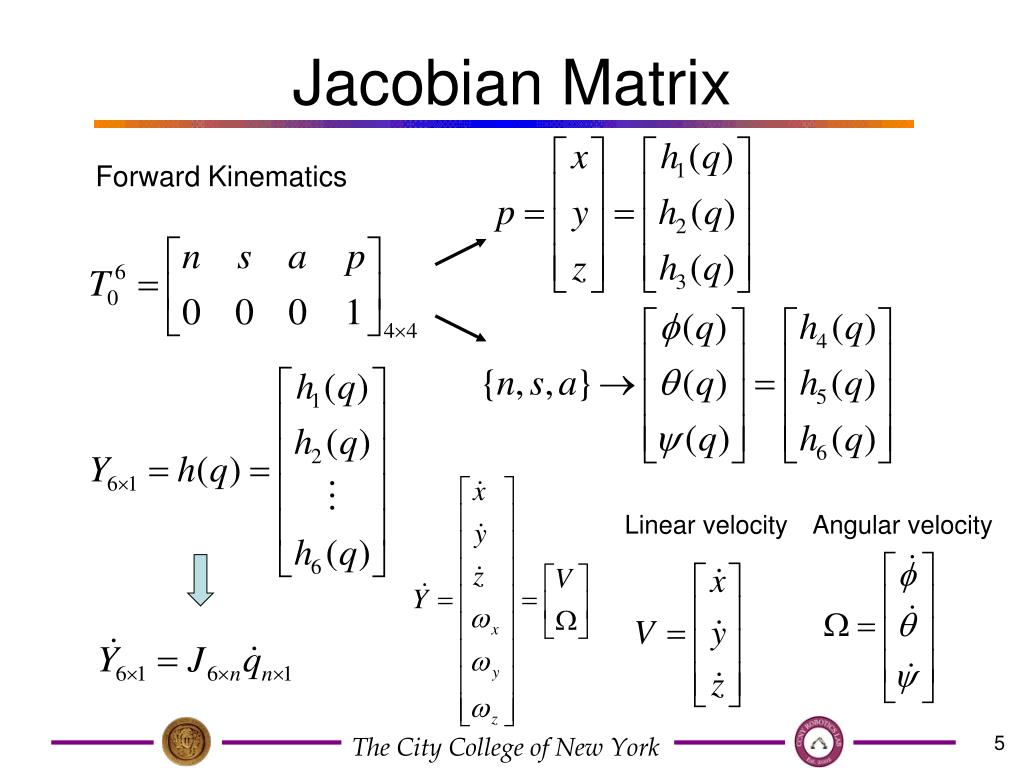
Jacobian matrix series#
When a Function Does Not Equal Its Taylor Series When Functions Are Equal to Their Taylor Series
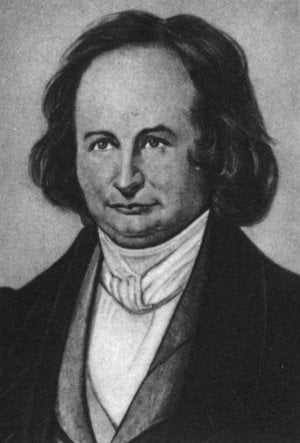
Strategy to Test Series and a Review of Testsĭerivatives and Integrals of Power SeriesĪdding, Multiplying, and Dividing Power Series Introduction, Alternating Series,and the AS Test Theorems for and Examples of Computing Limits of SequencesĬonvergence of Series with Negative Terms The process is then iterated until it converges. Each diagonal element is solved for, and an approximate value plugged in. Shifting the Center by Completing the SquareĪstronomy and Equations in Polar Coordinates The Jacobi method is a method of solving a matrix equation on a matrix that has no zeros along its main diagonal (Bronshtein and Semendyayev 1997, p. These concepts are named after the mathematician Carl Gustav Jacob Jacobi (18041851). Three kinds of functions, three kinds of curves function of a single variable, the Jacobian matrix has a single entry this entry is the derivative of the function f. Vector functions Jacobian: It is a matrix with partial derivatives of the input vector function Scalar functions Jacobian: For a scalar function, Jacobian.
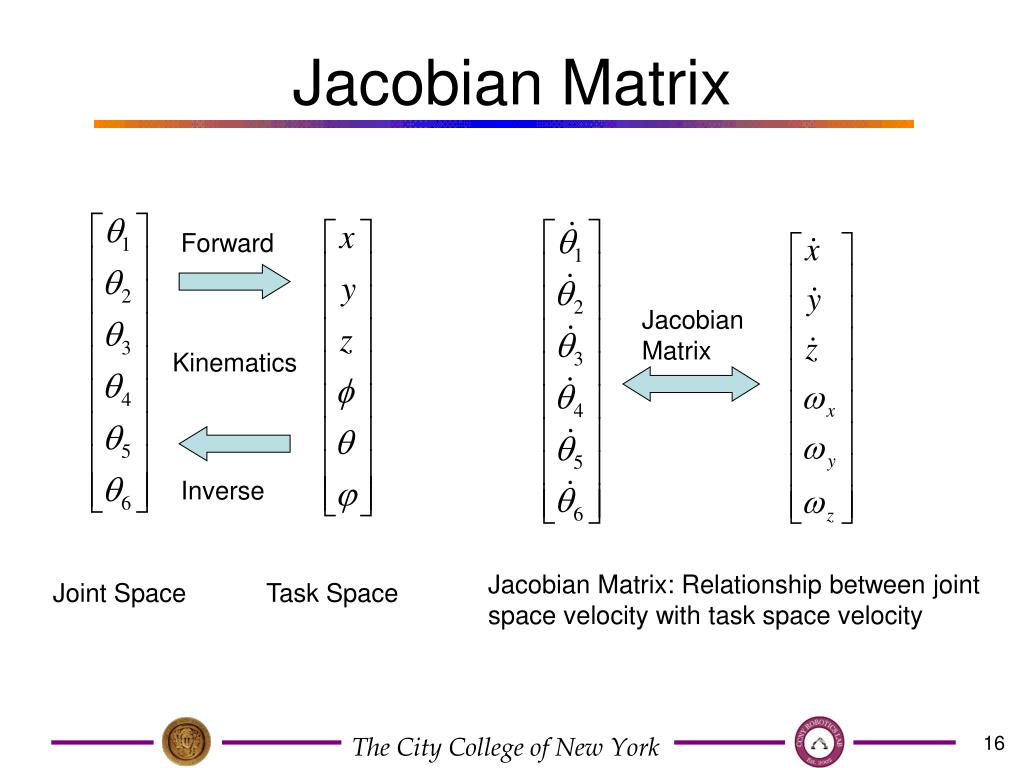
Type 2 - Improper Integrals with Discontinuous Integrands Type 1 - Improper Integrals with Infinite Intervals of It deals with the concept of differentiation with coordinate transformation. The main use of Jacobian is found in the transformation of coordinates. The matrix will contain all partial derivatives of a vector function. Jacobian is the determinant of the jacobian matrix. Improper Rational Functions and Long Division In reverse-mode AD, we are computing the jacobian row-by-row, while in forward-mode AD (which computes Jacobian-vector products), we are computing it column-by-column. Jacobian matrix is a matrix of partial derivatives. Product of Sines and Cosines (only even powers) Therefore, the Jacobian is always between 0 and 1. Product of Sines and Cosines (mixed even and odd powers or only The Jacobian in the Finite Element vocabulary is defined as the ratio between the smallest and the largest value of the Jacobian Matrix determinant. The Jacobian matrix has M rows and N columns, so if it is taller or wider one way we may prefer the method that deals with fewer rows or columns.Integration by Parts with a definite integralĪntiderivatives of Basic Trigonometric Functions In reverse-mode AD, we are computing the jacobian row-by-row, while in forward-mode AD (which computes Jacobian-vector products), we are computing it column-by-column. There are exceptions to this rule, but a non-rigorous argument for this follows: \(M > N\)) then jacfwd is preferred, otherwise use jacrev. Jacfwd and jacrev can be substituted for each other but they have different performance characteristics.Īs a general rule of thumb, if you’re computing the jacobian of an \(𝑅^N \to R^M\) function, and there are many more outputs than inputs (i.e. It is implemented as a composition of our jvp and vmap transforms. Jacobian matrix is a matrix of partial derivatives. As you saw above it is a composition of our vjp and vmap transforms. We offer two APIs to compute jacobians: jacrev and jacfwd: Reverse-mode Jacobian (jacrev) vs forward-mode Jacobian (jacfwd) ¶
